Precision agricultural tools are especially important in Siberia and other regions that are risky farming areas. The use of a neural network will help to reduce losses and increase the yield of crops. Tomsk State University biologists and the IT company Syncretis have taught AI to analyze field fertility and crop condition from satellite images. The new tool will be available to Russian agronomists developing precision agricultural technologies.
"An interdisciplinary group consisting of scientists, radiophysicists, meteorologists, IT specialists, and a number of other experts is working on the project", says associate professor of TSU Department of Soil Science and Soil Ecology of the Biological Institute, Oleg Merzlyakov. "Our partner, Syncretis, an IT services provider and developer of innovative software products and services, with partial involvement of an engineer at the Department of Meteorology and Climatology of Faculty of Geology and Geography of TSU, Vladislav Chursin, created a neural network for automated monitoring of the state of fields based on the analysis of satellite images. In order for the AI to recognize different types of soils, it was necessary to develop a system of biomarkers: having scanned them, the neural network will be able to determine all the most important functional features of a particular area of the field."
Scientists spent two years creating the data library. The research was carried out in the TSU strategic project Global Earth Changes: Climate, Ecology, Quality of Life, supported by the program Priority 2030. Soil condition (humidity, temperature) and surface air were analyzed with sensors constructed by TSU radiophysicists. Additionally, soil scientists analyzed the condition of fields, selected and assessed soil samples in different climatic zones (for example, dry soil in Khakassia and humid in Tomsk Oblast), and identified correlations between the reflectivity of soils and crops and their satellite images.
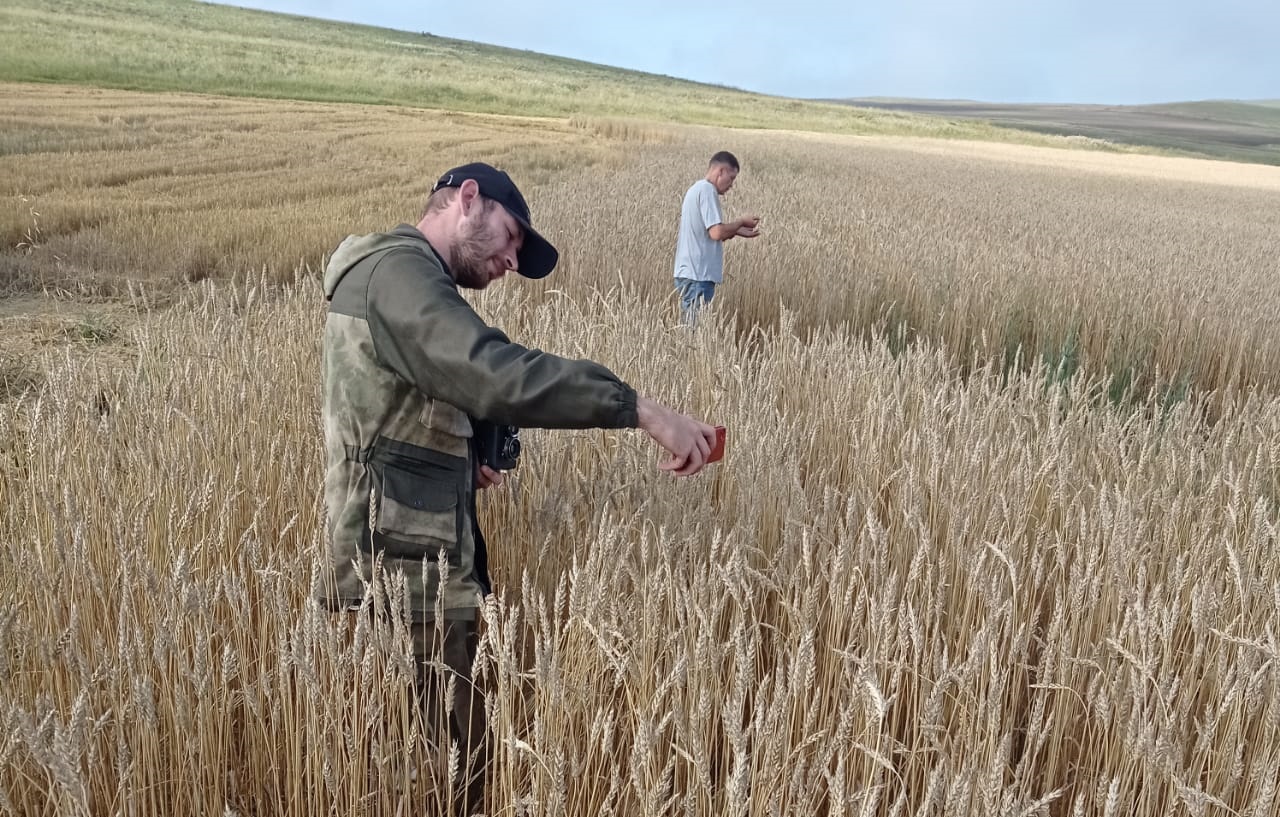
Using the data library and machine learning, Syncretis and Tomsk State University scientists were able to train the tailor-made neural network. Currently, the computer model is capable of identifying imperfections in the fields, determining the level of fertility from satellite images (especially, the concentration of humus), providing forecasts on possible damage to crops and establishing the cause (frost, drought, pests, disease), and offering recommendations for problem solving.
"For example, if the damage is caused by a pest, the neural network outlines the problem area," explains Oleg Merzlyakov. "Next, the problem is solved with the help of the TSU Faculty of Innovative Technologies staff and their drones, for which they develop intelligent add-ons. At the first stage, drones carry out additional examination of the terrain, then the spot gets treated with maximum precision using the necessary agent. The lack of micro- and macro-elements is solved in the same manner. There is usually no need to process the entire field. The neural network determines the location and the shortage of elements, and drones execute precise treatment, which helps the agricultural producer save money."
As of now, developers are determining which platform the new digital service will be hosted on. It will be available as a service to Russian agronomists working with precision farming tools and technologies.
Moreover, TSU scientists are developing a supplementary educational program, Digital Agronomist, for specialists who have basic specialized education but aspire to master the use of digital technologies in agriculture (coordinator of the program is Irina Kuzhevskaya, associate professor of the Department of Meteorology and Climatology of the TSU Faculty of Geology and Geography).
Testing of the automatic monitoring system for the condition of fields will be conducted in 2023, on the fields in the Novosibirsk Oblast belonging to an industrial partner in the TSU strategic project Engineering Biology, with the support of the program Priority 2030.